Version 2023 introduces significant improvements to the Classic algorithm for pKa prediction in PhysChem Suite which also impacts other predictions on the Percepta Platform. The updated algorithm increases the accuracy of prediction for broader chemical space than the previous algorithm and offers significant increase in calculation speed—also impacting other prediction models. Read below for details, and contact us for help upgrading your software.
Increased Speed of Calculation for “Apparent pKa”
You will notice up to a 5–10-fold increase in the speed of calculation of Apparent pKa values—the pKa value equivalent to that observed experimentally. This will be particularly noticeable in batch calculations and for compounds with multiple ionizable centers. The improved performance is a result of:
- A new method for calculation of apparent pKa where one preferred protonation/deprotonation pathway is chosen for the calculation
- Optimization of calculations for pKa micro constants
Predict pKa More Accurately for Expanded Chemical Space
You can have greater confidence in calculations from a significant expansion of the pKa Classic algorithm training set. Improvements comprise:
- Inclusion of ~4000 environmental, pharmaceutical, and chemical compounds to the training set
- An increase in the number of experimental pKa values to more than 40,000
- Rebuilding of existing Hammet-type and new equations for novel chemical classes
- Addition of new ionization centers
The linear regression scatter plots below (Figure 1) show the comparison of experimental versus predicted pKa, before and after training, for a dataset of 373 pharmaceutical compounds with 578 ionization centers. The average error of prediction is significantly lower in v2023 (0.26 log units versus 1.48 log units in v2022), and R² is closer to 1 (0.98 versus 0.52 in v2022). Three ionization centers were not predicted by the v2022 algorithm.
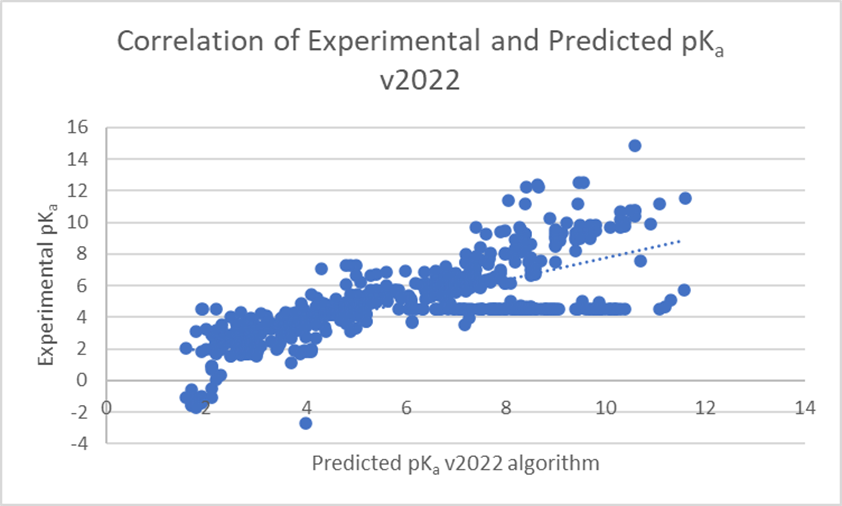
Training of the algorithm resulted in significant improvements in prediction accuracy for these 373 novel pharmaceutical compounds (see Figure 2). 84% of pKa values for these compounds are now predicted within 0.5 log units (99% within 1 log unit). This compares to 38% within 0.5 log units (54% within 1 log unit) with three values not predicted at all with the v2022 Classic algorithm.
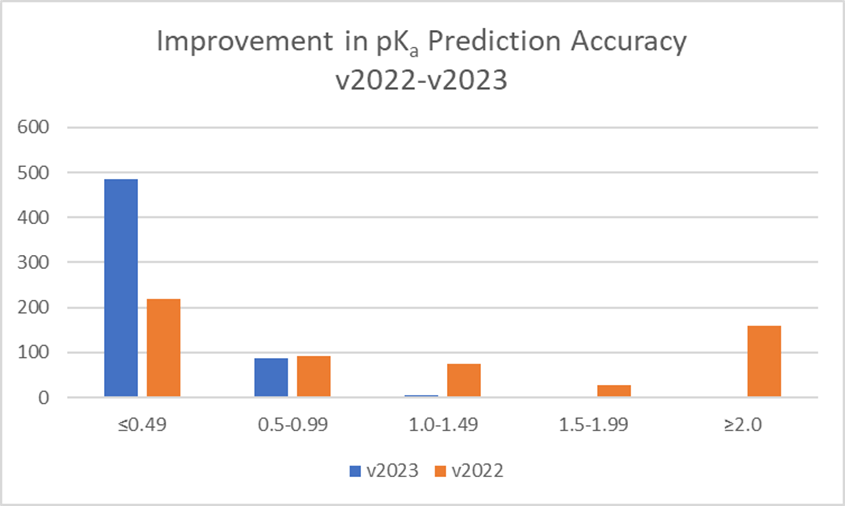
Compare Acidity/Basicity of Ionization Centers More Easily
You can now choose between two methods for the calculation of pKa with the Classic algorithm.
- Apparent pKa—simulates observed ionization of a molecule in aqueous solution and takes into account the protonation states of other ionizable groups at the relevant pH. (Default in previous versions.)
- Single pKa—a theoretical estimation of pKa for a single ionization center as though it is the only ionizable group in the molecule. All other protonation/deprotonation sites are assumed to be neutral.
Improvements to the pKa Classic algorithm directly impact predictions of logD and pH-dependent aqueous solubility. You can now expect greater accuracy for these calculations and expanded chemical space coverage.
Create Batch PDF Reports
You can now generate batch PDF reports from each module in PhysChem Suite, for all molecules in your active project. Previously, batch reports were only available from the Spreadsheet, including the values available there.
Unicode Support
You can now store textual data, written in your local language, in the Spreadsheet.
ACD/Labs’ development team is eager to collaborate with organizations to improve predictions for novel compounds. Do you have accurately measured experimental values for the predictions we support? Contact us to discuss how we may work together.
You Might Also Be Interested In...
ADME Suite
Version 2023 introduces a significant expansion of the training library for the Metabolic Stability calculator in ADME Profiler, better accuracy of underlying physicochemical property predictions in ADME Suite, and more.
Read moreTox Suite
Version 2023 introduces improvements in the hERG Inhibition prediction module, better accuracy of underlying physicochemical property predictions in Tox Suite, and more.
Read more